
How Explainable AI Will Transform the Banking Industry
In this blog, we outline a few banking use cases of Explainable AI and discuss why we believe it’s poised to transform how banks build and provide financial services.
In our recent blog, we examined what Explainable AI is, as well as the history of artificial intelligence.
The Need for Better Artificial Intelligence
Late last year, Apple came under fire for gender bias with the rollout of their new credit card (a joint venture with Goldman Sachs). Numerous reports detailed how two applicants with the same financial history were being offered significantly different interest rates and credit limits, which appeared to be driven solely by their gender. From Forbes:
Wozniak went on to call for government intervention in the matter, citing “excessive corporate reliance on mysterious technology” according to a report from Bloomberg. According to Wozniak, “These sorts of unfairnesses bother me and go against the principle of truth. We don’t have transparency on how these companies set these things up and operate.”
Whether this was a conscious decision or not, it’s clear that Apple and Goldman Sachs had built an algorithm that displayed an unnecessary and potentially illegal bias. With traditional “black box” AI systems, it would be very difficult for a bank to analyze and understand where this bias originated. With XAI, this becomes much easier to solve.
Why is Explainable AI Valuable for Banks?
Let’s do a thought experiment: Due to the significant economic impact of COVID-19, your manager is concerned about how customers will be able to make payments on their loans in 2020. You decide to engage with your engineering team to design an AI system that predicts people who will likely default on their loans in the next 6 months. In order to not lose your customers’ business, you decide to proactively reach out to them with an offer to restructure their loans to interest-only for two years, thereby lowering their monthly payments in the short-term.
Your engineering team has built the system and outputs a list of customer names. Are you ready to launch your strategy? Likely not. You probably want to validate how the AI system arrived at that conclusion before making the impactful business decision to restructure the set of loans potentially unnecessarily. With many AI systems, you would be unable to validate how the AI system arrived at its list of customers and instead have to trust that you added the right inputs, and the engineers built the right logic.
In contrast, an Explainable AI system would allow you to understand and validate how the AI system arrived at its conclusions. Which system would make you more comfortable to make an important business decision?
Explainable AI Use Case
- Optimizing the Onboarding Process
Banks lose millions of dollars a year in lost revenue due to inefficient customer onboarding processes. For banking customers, they lose out on potentially business-saving loans when onboarding processes are inflexible.
This issue has been magnified during the COVID-19 pandemic as small business demand for loans spiked but many banks were unprepared to evaluate the health of businesses. XAI can help banks provide better digital self-service journeys, quickly conduct complex eligibility checks while adhering to bank risk criteria, and establish appropriate pricing models, all while maintaining transparency with customers and maintaining risk controls. Learn more about how we’re helping small businesses and retail customers access funding during the COVID-19 crisis with our XAI solutions.
2. Deliver Personalization at Scale
All User Experience (UX) experts struggle to figure out how to create personalized experiences at scale. For banks, they must make decisions about questions like:
- Which product(s) should we place at the top of the menu of our banking app?
- Which questions should we ask first in an application to reduce abandonment?
- Who (and when) do we target promotions to?
- When should a customer service representative proactively reach out to a customer?
Historically, UI/UX designers would run A/B tests to see which variation performed best most for the most amount of people and then built their app accordingly. But with the advent of AI and machine learning, there is no need to select only one variation—systems today can predict and personalize experiences for every single person, without the need of a UI/UX designer to consciously make that decision.
At Temenos, we believe there is a huge opportunity for XAI to handle this decision-making process and allow banks to create personalized experience for their customers at scale.
3. Limit Human Biases
Banking is an inherently error prone process when relying solely on human decision-making. Natural biases are present whenever value decisions are made, especially when extending lines of credit. Who should receive credit? How much should they receive? Can machines make better decisions than humans can by predicting default rates given hundreds of data points? Early AI applications have demonstrated that there is a very real danger of software actually enhancing human biases, specifically along the lines of race. With Explainable AI, it makes it possible for quality control engineers to detect where these biases may be accentuated so that banks can teach their algorithms to XAI products to recognize and eliminate those cases.
If you’re interested in learning more about Temenos’ AI solutions, visit our XAI product page or watch our on-demand webinar “Explainable AI—Not Just Desirable but Imperative”.
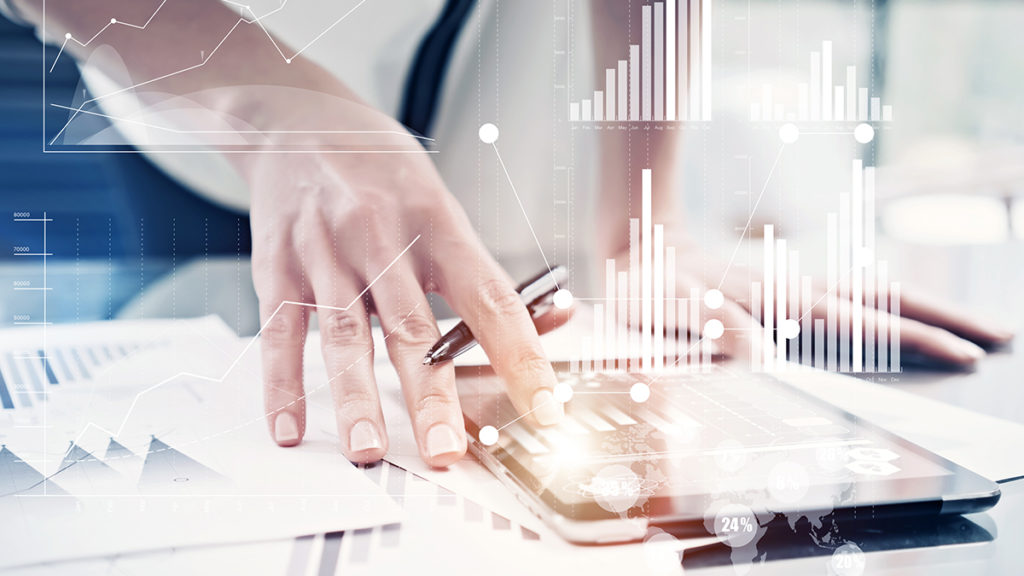